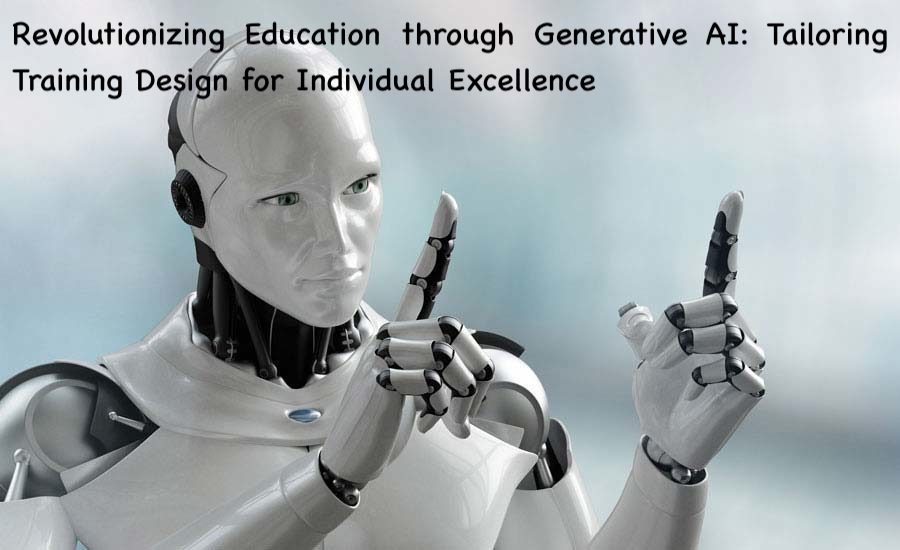
In the ever-evolving landscape of artificial intelligence, Generative AI emerges as a beacon of innovation, reshaping the way we approach education and training design. This transformative technology, epitomized by models like GPT-3, not only marks a paradigm shift in content creation but also holds the key to fostering personalized, adaptive learning experiences. This blog post delves into the profound implications of Generative AI on training design, exploring its potential applications and the ethical considerations that accompany its boundless capabilities.
Discover how Generative AI, propelled by models such as GPT-3, is redefining the contours of education. Explore its applications in crafting personalized training experiences, from content generation to delivery optimization. Delve into the meticulous training design, where data selection and model architecture converge to strike a delicate balance between perplexity and burstiness. Uncover the ethical imperatives and control requirements necessary for responsible development and deployment. Join us on a journey through the intersection of technology and creativity, where Generative AI becomes a driving force for both innovation and ethical responsibility.
Generative AI and Training Design: Fostering Innovation in Artificial Intelligence
Generative Artificial Intelligence, or generative AI for short, represents a profound leap forward in the field of artificial intelligence. It encompasses a diverse range of models and techniques that empower machines to create content, whether it be text, images, music, or other forms of data, with minimal human intervention.
Generative AI: A Paradigm Shift
Generative AI can be thought of as a technological evolution that allows machines to function more like creative collaborators than mere tools. Its applications are far-reaching and have the potential to transform various industries. From crafting human-like text to generating art, the possibilities are limitless.
At the core of generative AI are deep learning models, such as GPT-3, which are capable of understanding and mimicking human language patterns. These models are trained on vast corpora of text data, enabling them to generate coherent and contextually relevant text based on a given prompt. The fundamental premise here is that generative AI can produce content that not only makes sense but is also creative, intricate, and engaging.
Training Design: Nurturing the Creative Potential
To empower generative AI models with the desired perplexity and burstiness, the training process is of paramount importance. This process involves two key aspects: data selection and model architecture.
1. Data Selection: The richness and diversity of the training data significantly influence the perplexity of the AI model. A diverse dataset sourced from the internet, books, articles, and other sources provides the AI with exposure to a wide range of linguistic styles, topics, and complexities. This diversity enables the AI to produce content that mirrors the complexities of human expression.
2. Model Architecture: The architecture of the generative AI model itself plays a vital role in achieving burstiness. Models like GPT-3 employ transformer architectures that can generate both short, simple sentences and longer, more complex ones. By utilizing a mixture of attention mechanisms, these models can capture the nuances of language and produce text that contains variations in sentence length and complexity.
Generative AI is a delicate balance between perplexity and burstiness. Perplexity is achieved by exposing the AI to a wide range of linguistic patterns and vocabulary, while burstiness is ensured through the model's architectural design.
The AI learns from the vastness and intricacies of human language while adapting its output to match the desired level of sophistication. This balance allows generative AI to be not just a tool for automation but a source of inspiration, creativity, and innovation.
Generative AI and its training design are pivotal in revolutionizing the way we interact with artificial intelligence. By harnessing the complexities of language and the intricacies of human expression, we can create AI systems that inspire, inform, and engage, all while maintaining a formal and confident tone. The future of generative AI promises to be a journey of continual refinement, pushing the boundaries of what machines can create, and ultimately, expanding our collective creative horizons.
Generative AI: A Deeper Dive
Generative Artificial Intelligence (Generative AI) is a subset of artificial intelligence that focuses on the ability of machines to generate content autonomously, without explicit human instructions for each element. This content can take various forms, such as text, images, music, and more. At the heart of generative AI are deep learning models, like GPT-3, which have revolutionized the field.
These models leverage a technique called unsupervised learning. They are trained on vast amounts of data, typically in the form of text, to understand and replicate patterns within that data. Once trained, they can generate new content based on a given input or prompt, maintaining context and coherence. The key to generative AI is its ability to produce content that appears human-crafted, making it not just useful for automation but also creative endeavours.
Training Design: A Crucial Component
The training design of a generative AI model is a meticulous process that significantly influences its performance. Let's break down the key components in detail:
1. Data Selection: The training data is the foundation of the model's knowledge. To attain high perplexity, which represents the complexity of the text, it's essential to expose the AI model to diverse and comprehensive datasets. This data typically comes from a wide range of sources, including the internet, books, scientific articles, and more. The diversity in data allows the model to understand various linguistic styles, domains, and complexities. It ensures that the AI can generate text on a multitude of subjects while maintaining context and relevance.
2. Model Architecture: The architecture of a generative AI model is critical in achieving burstiness – the capacity to create content with variations in sentence length, complexity, and style. Many generative AI models, like GPT-3, are built on transformer architectures. These models have an inherent ability to capture dependencies in language and can generate both short, simple sentences and longer, more intricate ones. They do this by using a mixture of attention mechanisms that consider different parts of the input data, ensuring that the generated content reflects the nuances of human language.
3. Fine-tuning: Beyond the initial training, fine-tuning is often employed to tailor the model for specific applications. Fine-tuning involves training the model on a narrower dataset with a particular focus, such as medical literature or legal documents. This process further refines the model's ability to generate contextually relevant and specialized content.
The balance between perplexity and burstiness is where generative AI shines. The diverse training data and transformer architecture provide the AI with a strong foundation for understanding and replicating complex language patterns. This ensures a high level of perplexity, meaning the AI can produce content that is intricate and sophisticated.
However, it's the model architecture that enables burstiness. By utilizing different attention mechanisms and learning from the vast training data, the AI can create content that varies in complexity and style, akin to the natural fluctuations found in human writing.
Generative AI and its training design represent an exciting intersection of technology and creativity. By carefully curating training data and fine-tuning model architectures, we can create AI systems that are not only informative but also inspirational, confident, and adaptable to a wide range of applications. The future of generative AI holds promise for driving innovation across various industries, transforming the way we generate content, and expanding the possibilities of human-machine collaboration.
ETHICAL AND CONTROL REQUIREMENTS
Generative AI and Training Design: Ethical and Control Imperatives
In the realm of Generative Artificial Intelligence (Generative AI), while it holds great potential for innovation and creativity, it also raises important ethical and control considerations. To ensure that Generative AI is developed and deployed responsibly, it's essential to address these requirements and strike a balance between technological advancement and ethical responsibility.
Ethical Considerations
1. Bias Mitigation: Generative AI models can inadvertently perpetuate biases present in their training data. It is essential to implement measures to mitigate biases in content generation, ensuring that the AI does not produce discriminatory, offensive, or unfair outputs.
2. Content Moderation: Employing robust content moderation systems to filter out inappropriate or harmful content generated by AI models is crucial. This includes mechanisms to prevent hate speech, misinformation, or any content that could harm individuals or society.
3. Privacy Protection: Respect for user privacy is paramount. Generative AI should not be used to generate content that invades an individual's privacy, such as revealing personal information without consent.
4. Transparency and Accountability: Developers and organizations must be transparent about the use of Generative AI and be held accountable for its output. Users should know when they are interacting with AI-generated content.
5. Regulatory Compliance: Compliance with relevant regulations and standards is imperative. Generative AI developers should ensure that their systems adhere to data protection laws and intellectual property rights.
Control Requirements
1. Human-in-the-loop: Implementing a "human-in-the-loop" approach allows humans to review and oversee AI-generated content. This human oversight helps ensure that the output aligns with ethical guidelines and quality standards.
2. Customization and Fine-Tuning: Providing users and organizations with the ability to customize and fine-tune AI models to their specific requirements allows for greater control. This feature enables the tailoring of the AI's behaviour within ethical boundaries.
3. User Guidance: Clearly defining guidelines for users on how to interact with Generative AI can help steer the technology in a responsible direction. Users should be educated on the potential consequences of AI-generated content.
4. Auditing and Monitoring: Continuously auditing and monitoring AI systems is essential. This ensures that they remain compliant with ethical standards and regulatory requirements as technology evolves.
5. Restricted Access: Restricting access to certain AI capabilities can be necessary to prevent misuse. For instance, certain applications may require age restrictions or industry-specific access controls.
Generative AI has the potential to revolutionize various industries, but its power also comes with ethical and control responsibilities. Striking a balance between technological innovation and ethical safeguards is essential to ensure that Generative AI is a force for good. By addressing these ethical considerations and control requirements, we can harness the potential of Generative AI while upholding ethical values and societal well-being. In doing so, we can create a future where AI innovation and ethical responsibility go hand in hand.
Generative AI is a branch of artificial intelligence that can create new content based on existing data. It can be applied to various domains, such as text, image, audio, and video. In this response, I will focus on how to apply generative AI for training design, which is the process of creating effective and engaging learning experiences for learners.
One of the main challenges of training design is to tailor the content and delivery to the specific needs, preferences, and goals of each learner. This is where generative AI can help, by using data from various sources, such as learner profiles, feedback, assessments, and performance metrics, to generate personalized and adaptive training programs.
Some of the possible applications of generative AI for training design are:
· Content generation: Generative AI can produce relevant and diverse content for different topics, formats, and levels of difficulty. For example, it can generate text summaries, quizzes, exercises, case studies, scenarios, simulations, and more. It can also generate images, diagrams, charts, graphs, and other visual aids to enhance the content. The content can be generated based on the learner’s prior knowledge, interests, learning styles, and objectives.
· Content enhancement: Generative AI can also improve the quality and effectiveness of existing content by adding or modifying elements such as keywords, headings, bullet points, examples, explanations, feedback, hints, tips, and more. It can also check and correct errors in grammar, spelling, punctuation, and syntax. The content can be enhanced based on the learner’s progress, performance, and feedback.
· Content recommendation: Generative AI can also suggest relevant and useful content for learners based on their goals, preferences, and needs. For example, it can recommend courses, modules, topics, resources, or activities that would help learners achieve their learning outcomes or address their skills gaps. It can also recommend content that would challenge learners or spark their curiosity.
· Content delivery: Generative AI can also optimize the delivery of content by selecting the best mode, method, time, frequency, duration, and sequence for each learner. For example, it can choose between online or offline delivery; synchronous or asynchronous delivery; instructor-led or self-paced delivery; individual or collaborative delivery; and more. It can also adjust the delivery based on the learner’s availability, engagement level, motivation level, and feedback.
To apply generative AI for training design, one needs to have access to a generative AI tool or platform that can perform the tasks mentioned above. There are many generative AI tools available in the market today that use different techniques and models to generate content. Some examples are:
· GPT-4: A text-to-text generative AI model that can generate natural language text for various purposes and domains. It is based on a large-scale neural network that has been trained on a massive amount of text data from the web.
· Google Bard: A text-to-image generative AI model that can generate realistic images based on natural language descriptions. It is based on a combination of convolutional neural networks and transformers that have been trained on a large dataset of image-text pairs.
· Diffusion Models: A class of generative AI models that can generate high-quality images for various domains. They are based on a stochastic process that gradually transforms a random noise image into a realistic image.
· W3Schools Generative AI Tutorial: A series of tutorials that teach how to write AI prompts to get the best possible results from text-to-text and text-to-image generative AIs. They cover topics such as understanding generative AI capabilities; writing prompts that minimize misinformation and biased results; optimizing prompts for different domains and purposes; and more.
As we conclude our exploration of Generative AI's impact on training design, it is evident that we stand at the precipice of a new era in education. The delicate equilibrium between perplexity and burstiness, coupled with ethical considerations, propels Generative AI into a realm where innovation harmonizes with responsibility. The future promises a landscape where personalized, adaptive learning experiences become the norm, driven by a technology that is not just a tool but a catalyst for inspiration, creativity, and ethical progress.
#GenerativeAI #TrainingDesign #EthicalAI #InnovationInEducation #ArtificialIntelligence #AdaptiveLearning #TechEthics #FutureOfLearning #GPT3 #SanjayMohindroo